Completing this module will increase your understanding of what public health data are collected, how they are collected, and how they are used for planning and programming of health services, monitoring of services and health status, and evaluation of interventions. This module provides an overview of the types and sources of public health data. The strengths and limitations of the various sources of data are also covered, as these need to be considered when using data for planning and policymaking. Further explanation is provided on how public health data are used. Monitoring and evaluation of health programs are also covered, as these are important uses of health data.
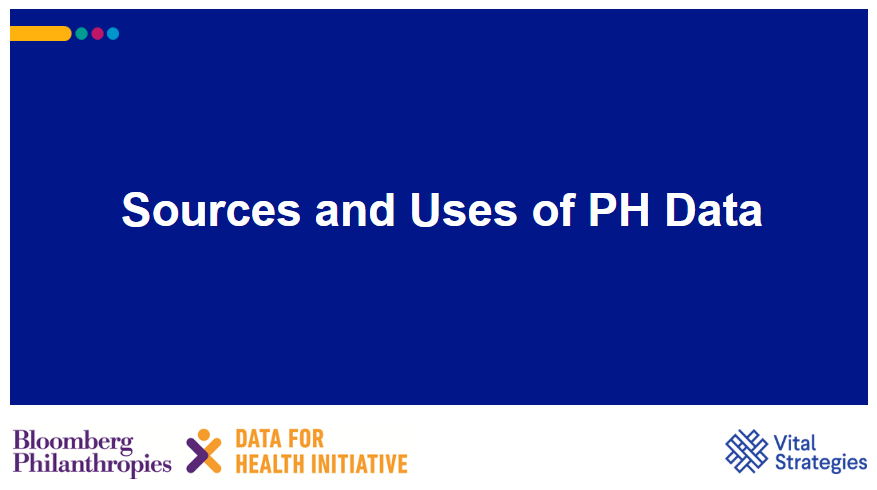
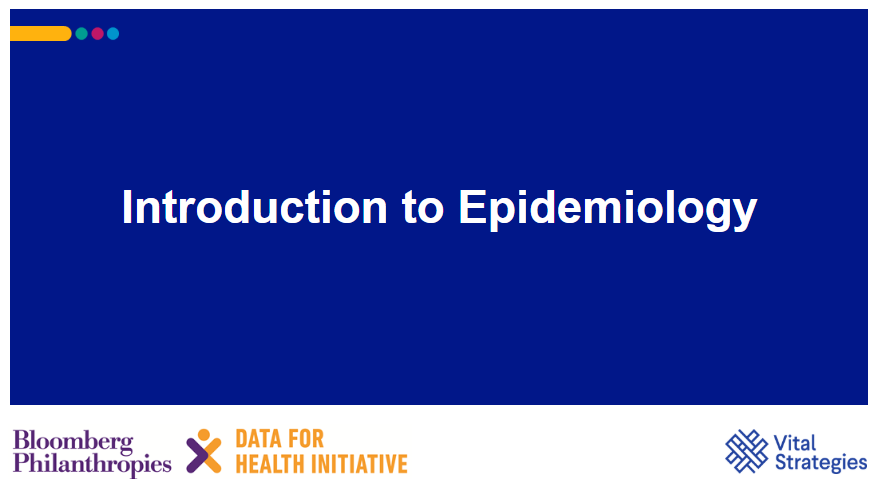
This module explores measures of association that are used to compare groups of different people, groups across different places, or one group over time. Age-standardized data is often required when making such comparisons. The module therefore also provides an explanation of when standardization of public health data is important and how to age-standardize data. It concludes by providing details on more complex measures of potential impact, including population attributable fraction and excess mortality. Completing this module will improve your knowledge of the importance of comparing groups in epidemiology, as this helps to identify populations most affected by poor health. The population attributable fraction is then used to estimate the reduction in disease due to the removal of harmful exposures or the implementation of beneficial public health interventions.
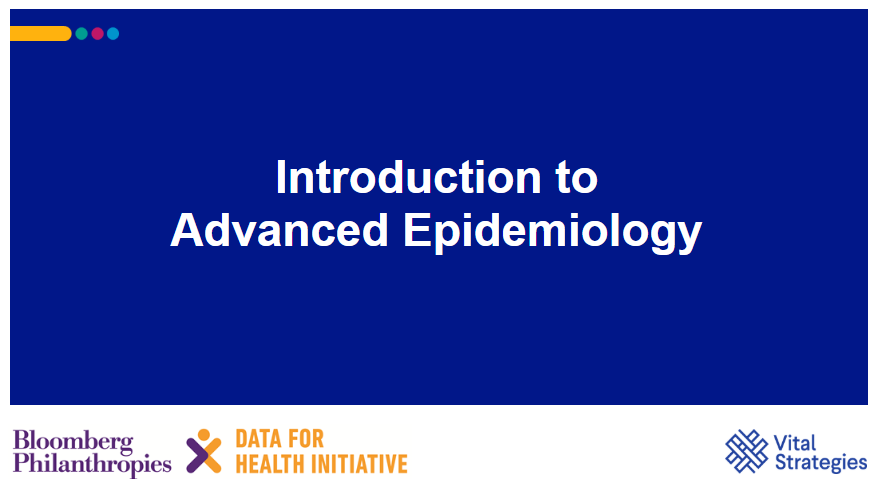
Understanding the social determinants of health and inequalities in a setting can help identify where health inequities exist, inform resource allocation, and tailor public health programs. This module begins with the social determinants of health and how they influence and affect health outcomes. Health inequalities and inequities are then described, and data from the World Health Organization Health Equity Assessment Toolkit (HEAT) are used to present and calculate measures of inequality. By completing this module, you will gain an understanding of health inequalities and inequities, and how to assess these for a given population.
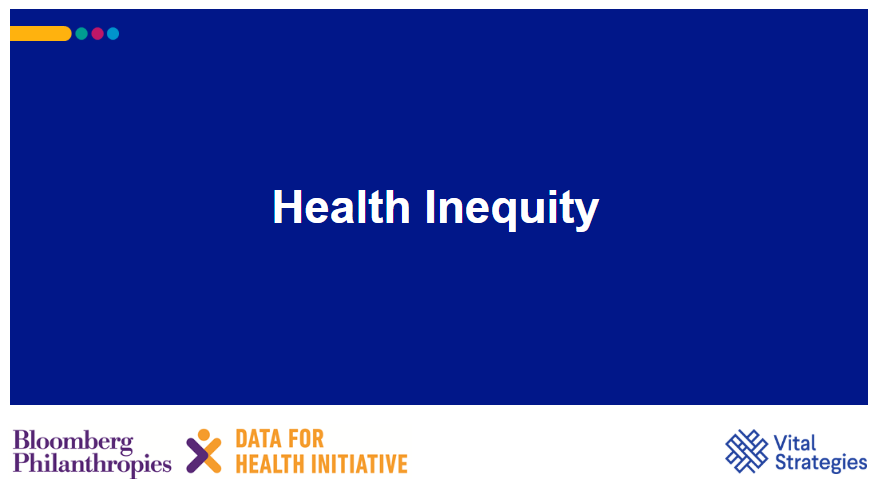
Large amounts of health data are collected by governments. Although some analysis of the data is usually done, the data are not always used in decision-making. This module provides an understanding of public health data and how it is used to inform public health planning, resource allocation, and policymaking. It provides government staff with a framework for improving the use of data for decision-making. Completing this module will help you develop a structured approach for making data-driven decisions to improve population health.
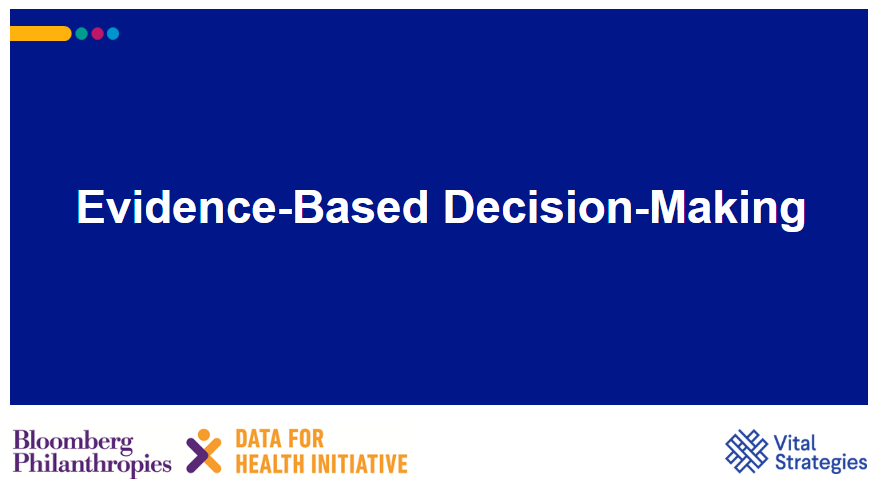
The goal of a visualization is to allow the data to tell a story. They can help to communicate a message to your reader that may be difficult to convey in a table. The module commences with the type of data stories that can be told, as this helps you define the message you want to communicate (e.g., change, comparison, composition, or correlation). The next step is to select the most appropriate visualization based on your communication purpose and the characteristics of your data. The module then covers the most important principles that should be considered when designing a visualization. The final section discusses the purpose of the maps and the types of maps that are used to share public health information. Completing this module will provide you with the knowledge of how to select and design the best visualization to tell your story.
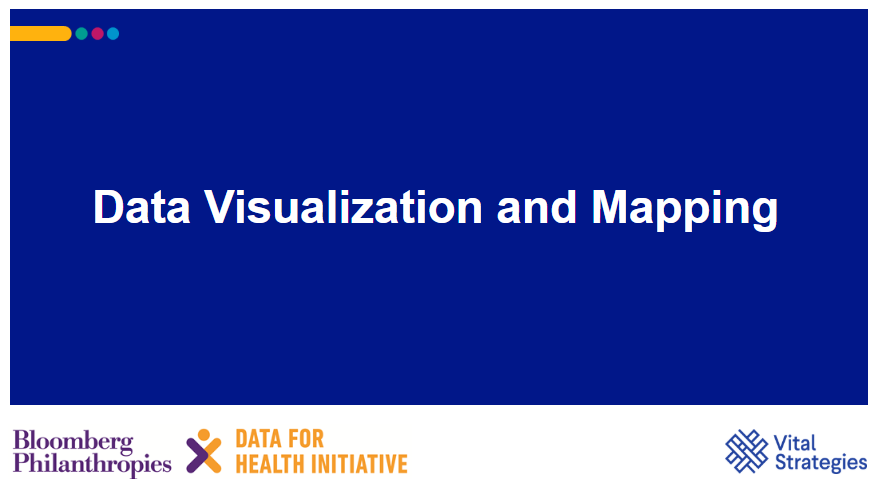
Measuring the burden of disease helps clarify the impact of a health problem in a particular population or setting. Summary measures, such as disability-adjusted life years, are used to estimate the burden of diseases on population health. This module will introduce the different measures, describe how they are calculated, and provide you with an understanding of their limitations. By completing this module, you will understand how to estimate the impact of a health problem by measuring the burden of disease when comparing groups.
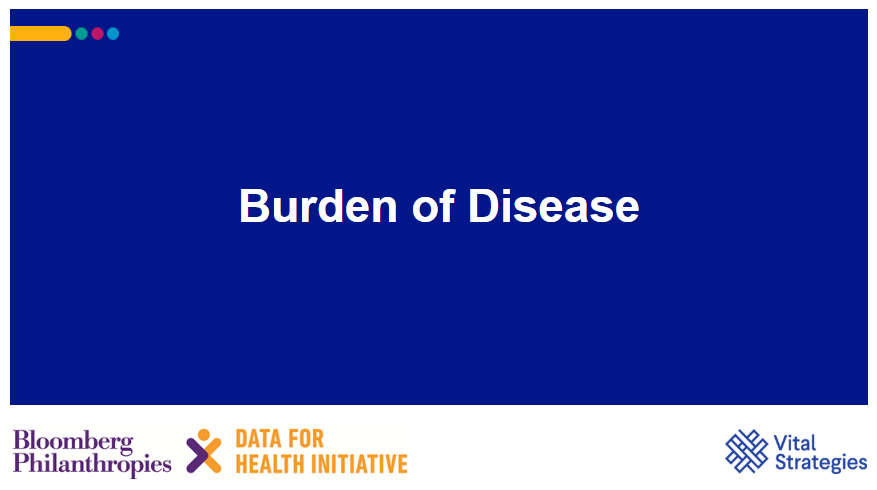
Data should not be analyzed without careful error checking and reflection on the quality. Incorrect assumptions or misinterpretations due to poor assessment of the data can result in a wrong decision or action, which could have detrimental health effects as well as potentially waste funds that could be used more fruitfully. The module provides you with an approach for assessing routine public health data and managing data quality issues.
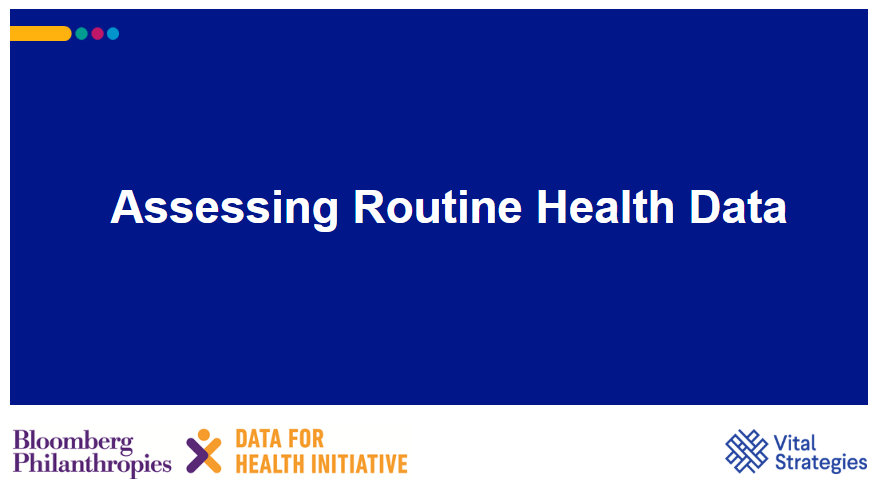